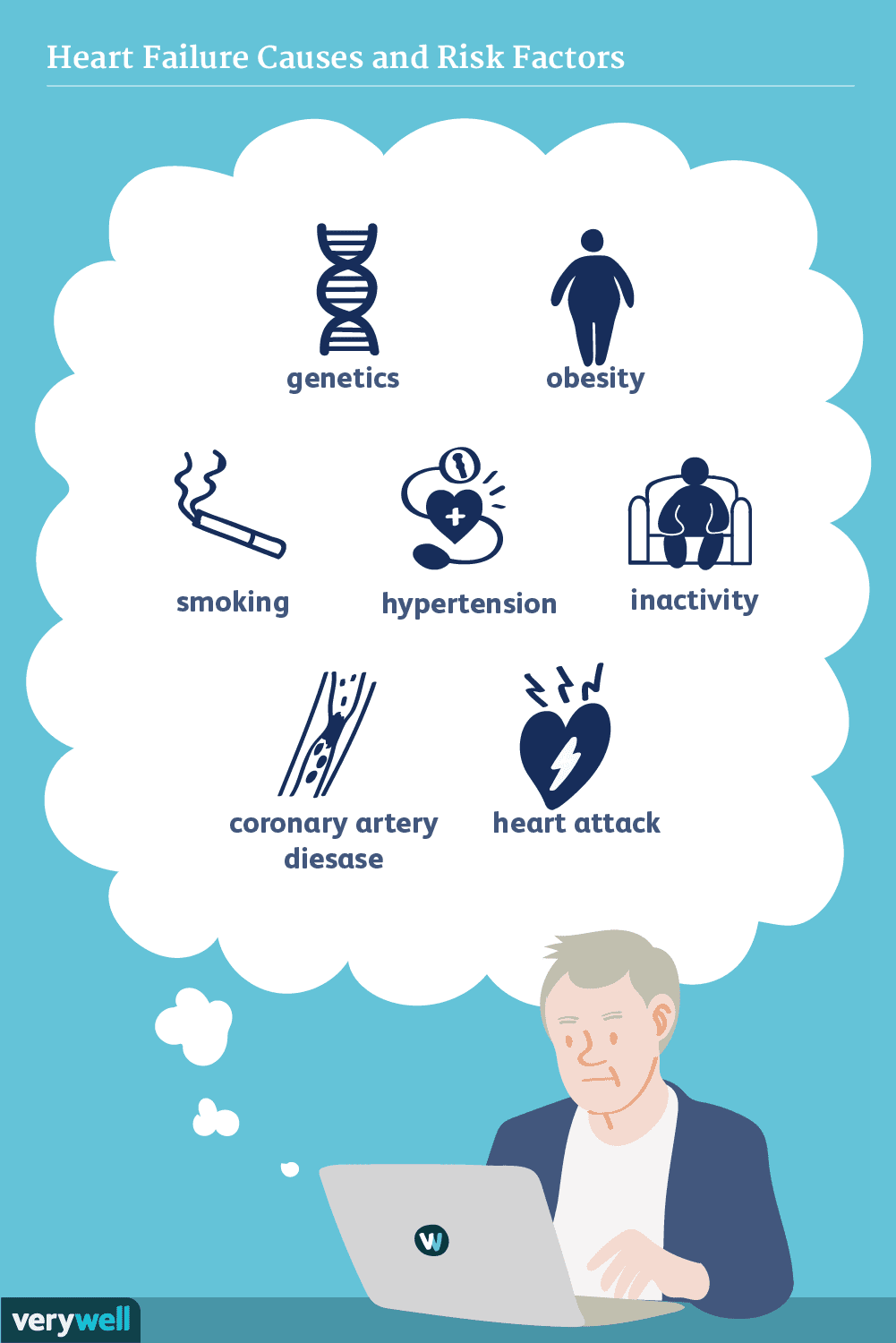
Mortality Risk in Heart Failure
Heart failure presents considerable mortality risks, highlighting the need for reliable predictors to support effective patient prognosis. This study explores the predictive power of specific clinical indicators, including ejection fraction, kidney function, smoking status, and follow-up time, for mortality in heart failure patients. Using a balanced training dataset, we conducted feature selection based on validation set accuracy, identifying these four indicators as the most influential subset. Models were subsequently tested on an unseen test set, with Random Forest achieving the highest accuracy at 87%, followed by Support Vector Machine (SVM) at 83.3%, and Logistic Regression at 80%. When all 12 initial indicators were applied, accuracy across all models converged to 83.3%. These findings emphasize the role of targeted feature selection in improving model performance, suggesting that focusing on key predictors can streamline clinical assessments while enhancing prognostic accuracy. This study underscores the potential of refined indica- tor selection to optimize resource allocation and improve patient outcomes in heart failure management.